报告题目:Reproducible, Reusable and Robust Data Science: Values, Challenges and Goals
报 告 人:Ana Trisovic 副教授 哈佛大学
照 片:
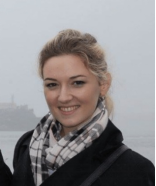
邀 请 人:李伟
报告时间:2020年12月21日上午9:00-9:45
腾讯会议ID:300 853 885
报告人简介:Ana Trisovic是哈佛大学定量社会科学研究所(IQSS)的斯隆博士后学者。她的研究主要集中于计算再生性、数据保护和数据科学,与Dataverse团队合作,研究了如何通过自动化、元数据和封装来促进研究数据和代码的再使用。此前,Ana Trisovic是芝加哥大学CLIR博士后研究员,在那里她与能源政策研究所(EPIC)和图书馆工作。她于2018年在剑桥大学完成了计算机科学博士学位,博士论文题目是“CERN LHCb实验的数据保存和再现性”。在欧洲核子研究所工作期间,她与LHCb合作、CERN开放数据和CERN分析保存小组一起工作。在她攻读博士学位期间,她是纽纳姆学院Muir Wood学者成员、申请到CERN博士生项目和谷歌Anita Borg纪念奖学金的获得者。
报告摘要:Data science and machine learning have produced some of the most innovative and essential results across the disciplines in the last decade. However, the nature of the research also creates significant challenges in result reliability and verification. Therefore, an important goal of data science is to ensure that a published result is reliable and robust, which is essential for research trustworthiness. Computational reproducibility is defined as obtaining consistent results using the same input data, computational steps, methods, and code. Ensuring reproducibility in machine learning can be quite challenging due to the data biases and uncertainties, model training, choice of feature variables, etc. This talk will elaborate on motivation, challenges, and opportunities in reproducible data science. We will see new results of a study where 2104 projects based on the R programming language were re-executed in a clean computing environment. Only about 56% of the files were successfully re-executed, showing how hard it is to reuse old code even when it is released with open access.