报告题目:Variational Inference for Functions and its Applications
报告人:贾骏雄 副教授 西安交通大学
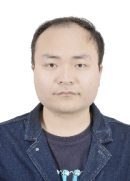
邀请人:冯晓莉 副教授
报告时间:2021年12月1日19:30
腾讯会议ID:342137355
报告人简介:贾骏雄博士2015年毕业于西安交通大学且于同年留校任教,2017年聘为西安交通大学数学学院副教授,主要研究领域为反问题的贝叶斯推断方法。主持国家自然科学基金青年、面上项目各一项,2017年获得陕西省优秀博士学位论文奖、陕西省数学会优秀论文奖,2018年获西安交通大学第四届十大学术新人奖,2020年入选陕西高校青年杰出人才支持计划。在SIAM Journal on Scientific Computing, Inverse Problems, Journal of Functional Analysis等国际著名期刊上共发表论文27篇。
报告摘要:In this talk, we will review the Bayes’ analysis method for inverse problems of partial differential equations (PDEs) and machine learning (ML), especially focused on the infinite-dimensional (ID) framework. Due to the computational efficiency, variational inference (a large class of efficient approximate sampling methods) achieves great success under the finite-dimensional (FD) setting in the ML community. We try to generalize the variational inference (VI) methods from FD to ID setting, which is more suitable for continuously formed inverse problems of PDEs and ML problems. Specifically, we first formulate the ID mean-field based VI and apply the proposed general framework to some linear inverse problems. Then, we construct the Stein variational gradient descent under the ID setting, which may be useful for solving nonlinear inverse problems. At last, combining the ideas of generating deep neural networks, we build a generating inference framework named VINet (variational inverting network) based on a detailed analysis of VI method in ID case, which shown to be a promising method for solving inverse problems of PDEs. Overall, three attempts for constructing VI methods in ID space have been shown, which indicate the usefulness of the ID spaced VI methods.
主办单位:环球360会员登录